- Nothing Ventured
- Posts
- Search Engines and the Time to Knowledge
Search Engines and the Time to Knowledge
Does generative search make us smarter?
Summary
“Where is the knowledge we have lost in information?”
A search engine’s value is based on how quickly it moves us from Information to Knowledge. The difficulty of that feat is dependent on the types of question we ask. Google’s page rank has solved for “informational based” or “closed ended questions.” Generative search platforms are attempting to reduce Time to Knowledge for all other question types.
Time to Knowledge for open-ended questions is largely dependent on the inquirer’s prior familiarity with a topic. Their context. A well-formed questioned is half-answered, but it takes a decent amount of time to understand what questions are even important to ask.
When we finally do arrive at some semblance of knowledge, the path there is often unauditible. I can’t attribute the value to any one search product. This places a limit on what generative search, in its current form, can charge as a subscription or monetize in the form of a compromised user experience (read: Ads).
In the below essay I explore a lot of these topics and also compare responses from four major generative search engines: Gemini, ChatGPT, Perplexity and Consensus.
Essay
“Where is the wisdom we have lost in knowledge, where is the knowledge we have lost in information?
These are lines from T.S. Eliot’s “Chorus from The Rock,” written in 1934. Although Eliot was no technologist, his writing often reflected concerns about industrialization and the growing complexity of human knowledge.
At the time, the world was emerging from World War I. Staring at the horrors of chemical warfare, intellectuals were skeptical of unchecked scientific progress. Many writers felt threatened by new communication technologies. Radio had become the dominant medium of information transmission and early experiments in Television were taking place. Alan Turing would propose his famous “machine” only two years later.
Amid such rapid technological change, Eliot laments that, in the modern world, we accumulate vast amounts of information, but fail to convert it into useful knowledge. Even when we do, we often fail to reach true wisdom.
Half a century later, the relationship between information, knowledge and wisdom was sterilized by organizational theorist Russel Ackoff; and portrayed in management textbooks as “The Knowledge Pyramid.”
At the time, the personal computer revolution was in full swing (IBM PCs, Apple Macintosh) and the early internet was evolving, hinting at a future of global information-sharing. It was a decidedly more optimistic age.

Eliot’s portrayal of losing something, of spiraling downward is directly contrasted with Ackloff’s illustration of transcendence. The information age reinforced the concept that technology could help you move up the knowledge pyramid rather than get mired in the ever-increasing information shit-pile.
Internet 2.0’s promise was the speed in which you could move up the pyramid. The speed of answers to our questions became the currency of every search technology. The speed at which you transcended from information to knowledge became the consumer value proposition. The “time to knowledge.”
This “Time to Knowledge” is largely based on the types of questions you are asking. For question categorization, I prefer to use the Purpose Based framework. There are four major question types: Informational (seeking to know), Exploratory (seeking to investigate), Diagnostic (seeking to analyze), and Evaluative (seeking to judge). Informational is a closed-ended question type, while all others are open-ended question types.

Google’s page rank algorithm has largely solved this problem for informational questions. These are the ones that naturally lend itself to key word search. This is going to blow your mind, but: Asking informational questions gets you…information as an answer (I know, right?!)

The “time to knowledge” on “informational” questions has been reduced to milliseconds. It has given Google the right to earn $175BN in ad-revenue annually.
What OpenAI is trying to accomplish with its GPT models, or Google with Gemini, or Perplexity is answer all of the open-ended categories accurately. It is a dramatically larger and more difficult problem space.
The “time to knowledge” with open-ended questions is largely dependent on the inquirer’s prior familiarity with the topic. Their context. A well-formed questioned is half-answered, but it takes a decent amount of time to understand what questions are even important to ask.
The power of generative search is the new found opportunity to ask the smartest person in the world stupid questions. There is no risk of retribution. No cause for embarrassment. Through these stupid questions, we establish a better understanding of a topic. The composibility of knowledge allows us to ask increasingly savvy questions.
The risk with generative search is that we ask the weighty questions first, with no prior knowledge-building and accept the first result as bible. We have rearranged the information layer with no movement up to knowledge. This is T.S. Eliots nightmare. We would have burned countless hours, compute time, mental and physical energy to get to a false certainty.
The power demands of these models have reignited discussions around nuclear fission, an energy source with the most terrifying tail risk in human history. To do so in exchange for mere information rearranging would be a double tragedy.
Testing generative search engines
To illustrate with a live example, I ask a very broad and weighty question to four popular generative search engines. This is an embarrassingly simplistic query, one that could be interpreted in countless ways by a human. It also happens to be one of the most complex question types; an Evaluative question:
Question: Do we really need more Nuclear Energy?
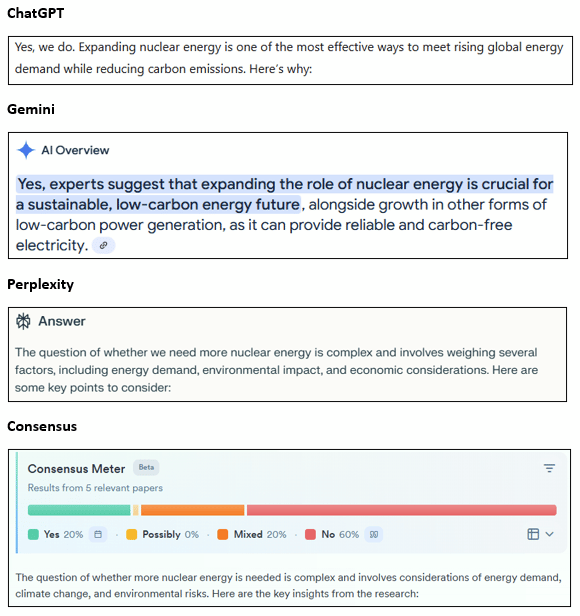
Suppose I were to trust the results blindly and form my view solely based on outputs; I would walk away with false certainty from ChatGPT and Gemini. A confident, binary “affirmative” response is not what we want from these models.
Perplexity at least hints at the nuance of an effective answer.
Consensus continues to design a search product that has the ethos of knowledge building. The citation of academic papers, potential for education on the relative strength of study design, and the avoidance of a falsely confident “affirmative” response all lend itself to further inquiry. Consensus gives you the greatest chance to move up the Knowledge Pyramid.
In reality, I spent months doing research on Nuclear Energy. I have a 50 page report (not generated by Deep Research) that I have shared with my internal team. I finally felt confident to even broach the topic with external experts.
The challenge is that, at the end of this research sprint, I could not attribute the new-found knowledge to any one search engine or source. I had taken bits and pieces of outputs from all four models. I had saved down academic studies. I had conducted primary research interviews.
My path to knowledge was unauditable
In such a world, how am I to evaluate a monthly subscription to any one search engine? If I cannot effectively attribute “time to knowledge” to any product, I am unclear on my true willingness to pay as a consumer.
Ultimately, the most impactful contributor to my knowledge on Nuclear Energy came from a book I randomly took out of the library.
Strange Glow: The Story of Radiation by Timothy Jorgensen, Chapter 16
In it, Timothy Jorgensen, a Professor of Radiation Medicine, takes the time to explain how scientists measured the historical impact of the world’s worst nuclear accidents, the ways scientists estimate risks of future accidents, and the limitations of doing so in complex systems. It is a 300+ page book written by an expert that has a goal of educating others.
Would I ever go back to a world where I am confined to taking books out of the library? Absolutely not. But, the book cost me 20 dollars and got me up the knowledge pyramid more effectively than any individual search engine. It illustrates that there are limits to consumer spend on generative search.
More comprehensive responses like Deep Research merely compound the problem. Without proper context, the 20 page research report is more output, but not better output. It is a potential distraction, information rearrangement masquerading as knowledge. We never internalize anything.
Philosophically, what I was trying to do throughout this entire research process is pinpoint the “why” behind a subtle, but persistent unease about the recent enthusiasm for more nuclear energy.
The first time I asked the question “Is more Nuclear Energy really necessary?” the machines had no context for such bias. They did not nudge me to refine my query by diving into what exactly I am afraid of (hint: nuclear contamination via spent fuel storage). They did not educate me on how scientists measured increased cancer incidence in the wake of Chernobyl. All of these things would have contributed to better questions and ultimately a more nuanced view on Nuclear Energy.
Which is why the race to better context is now of the utmost importance for Generative Search engines.
More on that in the next post.
Reply